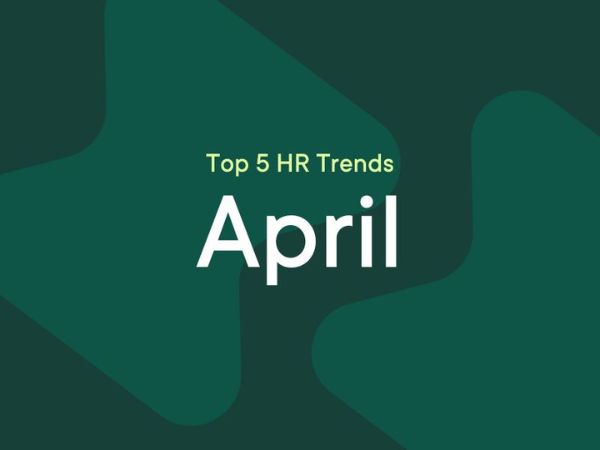
Give your people a voice with a tailored Xref Engage survey.
Increase retention and reduce turnover with quick employee feedback from an Xref Pulse Survey.
While it can be a scary thought to consider the role of automation and Artificial Intelligence (AI) in the context of work and jobs, it’s important to take comfort in the fact that there will always be people needed to support tech and guide it in the right direction.
In addition, a robot can never replace the intricacies and delicacies of human interaction. This is good news and a sigh of relief for HR professionals worldwide. However, the usage of AI and Machine Learning is growing, and it will support recruitment and people and culture teams to increase efficiencies and reduce manual work. Here’s how.
Artificial Intelligence (AI) and Machine Learning (ML) are two related technologies that have the potential to revolutionise HR. A number of organisations have already begun to integrate AI into their HR departments. In the 2019 Artificial Intelligence Survey, technical research and consulting firm Gartner discovered that 17% of organisations used AI-based solutions in the HR department. The same report indicates that another 30% of respondents planned to integrate AI in their HR department by 2022.
The Institute of Electrical and Electronics Engineers (IEEE) ‘Impact of Technology in 2022 and Beyond’ survey identifies AI and Machine Learning as the most important technologies of 2022. 51% of survey respondents also indicated that AI and Machine Learning are one of the primary technologies they plan to adopt in the next one to five years.
The success of any business is underpinned by how efficiently and effectively people, processes and technology combine. AI and ML are helping to automate many time-consuming administrative tasks, and the HR industry is definitely embracing the technology.
A 2022 Tidio survey of 1068 hiring managers and other HR professionals found that an overwhelming 95% of respondents think that AI will help in the application process. Around 68% of the survey respondents believe that AI will help reduce or eliminate unintentional bias in the recruitment process.
Now the question remains, what exactly is Machine Learning, and how can this technology be of help to HR professionals?
Artificial Intelligence, as a broad category, is the science of creating systems that solve problems in a manner similar to humans. Machine Learning is a field of Artificial Intelligence that revolves around machines being able to imitate human behaviour without being “smart”.
People interact with Machine Learning systems on a daily basis without knowing it. Chatbots, such as automated help chats on product pages, predictive text on your phone, the recommended movies on Netflix and more, are all examples of ML.
Rather than being programmed with strict information or actions, a Machine Learning algorithm is instead trained on large data sets. The algorithm detects data patterns and makes predictions or decisions based on these patterns. The more data available to the Machine Learning system, the more patterns arise, and the more accurate the predictions may become.
Take recommendations on Netflix as an example. The Machine Learning system in the streaming service keeps track of the movies and TV shows that you have watched. It makes a virtual database of descriptive keywords, genres and actors that have appeared in your watched list. It then makes predictions for what you might want to watch next based on that criteria.
The more content you watch on Netflix, the more data the recommendations system has to draw from. As a result, it becomes progressively better at finding shows you will probably like.
Users can also indicate whether they liked or disliked content. These choices improve the quality of information being used to train the algorithm, which improves the quality of recommendations.
The main problem with Machine Learning is the data that the system is trained on. As the old saying goes, “garbage in, garbage out”. This is especially true of ML projects. If the system is trained with poor quality or biased data, then what it learns will be of poor quality or biased.
Some of the largest failures in modern AI are due to the datasets being used to train algorithms. The data was identified as being of poor quality, incomplete or biased in some way.
HR professionals know that pre-screening, recruiting, and onboarding new staff dictates that a mountain of data needs to be processed. As a result, it can be easy for bottlenecks in the hiring funnel to emerge. This is especially true when there are large numbers of applicants or vacancies. Machine Learning has the potential to automate communications between candidates and employers, real-time hiring process alerts and document tracking.
Data collected from both current employees and job applicants can be used to train AI to perform or aid ina huge number of tasks within the HR space. Tasks include sourcing candidates, analysing the satisfaction and commitment of employees and predicting the risk of attrition.
A number of organisations, including Unilever, utilise Machine Learning to source talent. AI can be used to search social media, such as LinkedIn, to find resumes or profiles that fit the data sets the system has been trained with.
Such training can enable the algorithm to identify profiles and CVs of people that are ideal hires. Machine Learning can also be used to sort resumes submitted for a position, as long as the data being used is unbiased.
Chatbots can be used to replace initial contact between a hiring manager and a candidate. Chatbots can ask the candidates pre-set questions about skills, previous positions, salary expectations and so on. It can then analyse the responses, sorting the interviews into those that are high-priority second interviews and those that can be weeded out.
Chatbots may also be used as a valuable resource within an organisation. Uses include automating common HR queries and either answering questions directly or routing the query through to the correct HR professional.
Yes. In 2017, Amazon terminated its AI recruitment system, AMZN.O, which it had been working on for five years. According to many sources, including the American Civil Liberties Union (ACLU), the system couldn’t stop discriminating against women during the hiring process. The reason for the bias was the data used to train the system.
Amazon used 10 years' worth of resumes used when applying for jobs at Amazon. The idea was that the data collected would allow the AI to identify top candidates when resumes were submitted. This would leave only the final hiring decisions in the hands of humans.
The team didn’t take into account those who had traditionally applied for jobs in the tech sector when training the AI. The US tech sector is overwhelmingly male-dominated. According to Zippia, in 2022, 73.3% of the US tech sector is male, meaning that just over a quarter of positions are filled by women. As a result of the male-skewed data, the AI trained itself to downgrade factors attributed to women. It also overly promoted factors attributed to men.
The AI downgraded resumes with the word “women’s” in them. It discriminated against those with education from women-only colleges. It also favoured words such as “captured” and “executed”, which were apparently used frequently by male engineers.
AMZN.O may sound like a horror story that might turn anyone off the idea of AI in HR, but it’s not. It’s actually a fine example of the power of the data used to train machines. With good, unbiased data, AMZN.O could have been a revolutionary tool.
There is no quick or easy solution to training Machine Learning systems that could have saved AMZN.O. Machine Learning works in regards to the training data. When training AI technologies, the data being used and the Machine Learning model are key to everything.
There is no Machine Learning algorithm that is suitable for all purposes. Before any training can begin, the type of Machine Learning model being used must first be determined. There are two base models used for learning - supervised and unsupervised. Each has its pros and cons.
There is one main difference between the two learning methodologies. Supervised Machine Learning utilises labelled or curated training data while unsupervised learning uses raw or unlabelled data. Curating the data can remove the chance of the algorithm learning bias from the data. It can also run the risk of introducing human bias from the people labelling the data.
Unsupervised Machine Learning can’t be influenced by human-introduced bias, but runs the risk of learning bias. If a dataset shows that behaviour A correlated to outcome B then the algorithm can link or mix the two. In the case of AMZN.O, there are multiple examples of the correlation happening, such as fewer successful female candidates being intrinsically linked with women being unsuccessful.
The primary example was that resumes containing the term “women’s” in them were statistically less successful than those not containing the word. As a result, they were downgraded.
The bias towards male candidates in AMZN.O may have been avoided by removing any gendered language from the training data. In other words, changing from an unsupervised model to a supervised model.
No matter the ML model being used, the data it is trained with must be representative of the objective. This means the data should contain different groups and be inclusive. From an HR perspective, this means that the training data should be representative of both men and women in equal measure. That representation should be equally positive and negative.
Again using AMZN.O as an example, the data used was heavily skewed male in terms of both representation and success. If the data had contained a similar-sized pool of successful women it’s unlikely the bias towards male candidates would have been learned.
There is no instant fix for eliminating bias from Machine Learning, but there are a number of techniques that can be used to identify bias for elimination or remove bias from training data. Testing Machine Learning applications extensively before and after implementation can help identify problems before release or pick up any bias that may be introduced once the application is live.
As a Machine Learning application is only as good as the data used to train it, another method of eliminating bias is to use synthetic data for training rather than collected data. Synthetic data is based on a real dataset but is modified to be statistically representative of the desired outcome.
In the case of AMZN.O, synthetic data would ideally feature an equal number of successful and unsuccessful men and women, and no ethnic or racial information to eliminate any chance of bias creeping in.
In an effort to scrub bias from AI and ML, the European Union has proposed the EU Artificial Intelligence Act. This law, the first in the world by a major regulatory body, seeks to codify the training and use of AI and ML. One of the very specific purposes of the proposed law is that uses, such as CV-scanning to rank candidates must follow strict laws to prevent bias or discrimination.
Using Machine Learning to analyse large volumes of employees, HR can identify trends and opportunities. This can both streamline and improve a number of HR tasks.
The nature of Machine Learning and its ability to analyse and parse large amounts of data quickly makes it perfect for performing mundane, time-consuming and repetitive tasks. This makes it perfect for scheduling regular HR tasks, such as interviews, performance appraisals and meetings.
When properly trained, ML can be invaluable for identifying talent, either from submitted resumes or by data mining resources like LinkedIn. Effective candidate identification and sorting can speed up the recruitment process. It can also Identify candidates that may not have been obvious at first.
Machine Learning may be used to analyse and improve employee engagement through a number of methods. Using an AI/ML technology called Natural Language Processing (NLP) that analyses natural speech, HR can gain insight into employee sentiment. This can help to spot potential red flags that can be rectified before they lead to any employee attrition.
Machine Learning can be used to personalise employee training at scale. This enables organisations to better reskill or upskill employees by creating employee profiles for training. These profiles detail the skills they currently process and the skills they wish to possess as well as the style of learning they prefer. A system can then match the profile to the training needed to meet goals.
Predictive analytics is an advanced form of analytics that uses Machine Learning to analyse data patterns and predict possible future outcomes. It can be used in a number of ways by HR departments.
Using predictive analytics, organisations can identify employees who collaborate well to create effective teams.
On a larger scale, predictive analytics can be used to pinpoint what is working and what isn’t in an organisation. Analysing sentiment and performance and making predictions allows an organisation to take steps to improve mood and increase productivity.
Fears of Artificial Intelligence taking over HR are unfounded, as a human touch will always be needed when dealing with employees and ensuring the smooth operation of an organisation. That said, AI and ML will have a major impact on how HR professionals will perform their day-to-day tasks.
AI and Machine Learning can be incredibly powerful tools for HR professionals to use to streamline and improve HR workflow. From the use of chatbots to automate simple HR tasks to large-scale sentiment and performance analytics, ML can be utilised in a wide variety of ways.
The effectiveness of Machine Learning is dependent on the quality and volume of data used to train the Machine Learning model. Ensuring that the data is representative and unbiased can ensure that Machine Learning will be an asset to an HR department.